
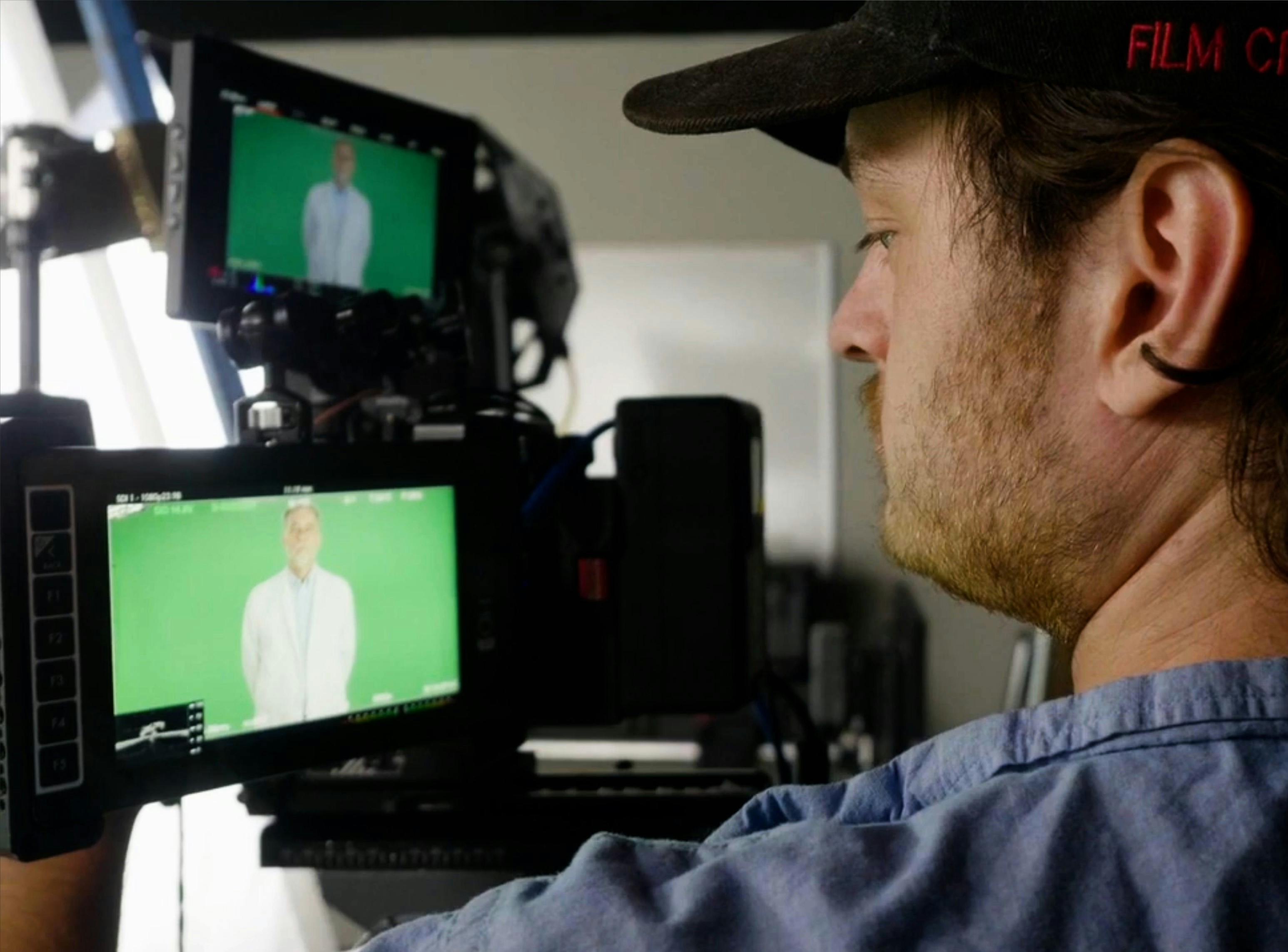
- #JINGPAI LABEL EXPERT INSTALL#
- #JINGPAI LABEL EXPERT MANUAL#
- #JINGPAI LABEL EXPERT LICENSE#
- #JINGPAI LABEL EXPERT PROFESSIONAL#
You will receive an email letting you know we received your application. Please note, incomplete applications will not be processed. We encourage you to upload your resume to your application so that all of your information stays together. If you’d like to be considered for a position, we request that you apply here. Learn more about what it’s like to be a Label Expert on our Company Culture page.
#JINGPAI LABEL EXPERT PROFESSIONAL#
Professional yet laid back, focused but not too serious, quirky. View Internship Opportunities Frequently Asked Questions
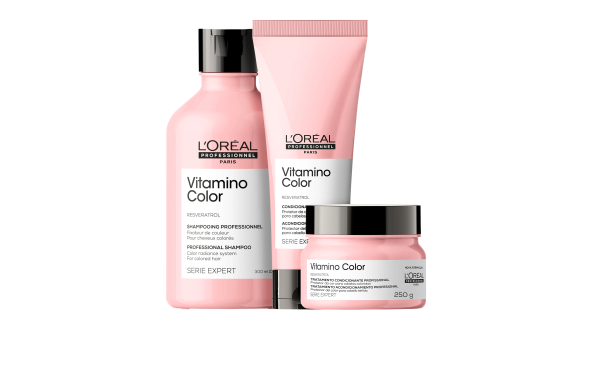
Put all reports in a csv file under the column name "Report Impression". This model differs from the one in our paper, which was instead trained on radiology reports from the CheXpert dataset. The MIMIC-CXR reports are deidentified and do not contain PHI. This model was first trained on ~187,000 MIMIC-CXR radiology reports labeled by the CheXpert labeler and then further trained on a separate set of 1000 radiologist-labeled reports from the MIMIC-CXR dataset, augmented with backtranslation. The batch size by default is 18 but can be changed inside constants.py Checkpoint downloadĭownload our trained model checkpoint here. You can control which GPU's are used by appropriately setting CUDA_VISIBLE_DEVICES.
#JINGPAI LABEL EXPERT INSTALL#
(Recommended) Install requirements, with Python 3.7 or higher, using pip.īy default, all available GPU's will be used for labeling in parallel.
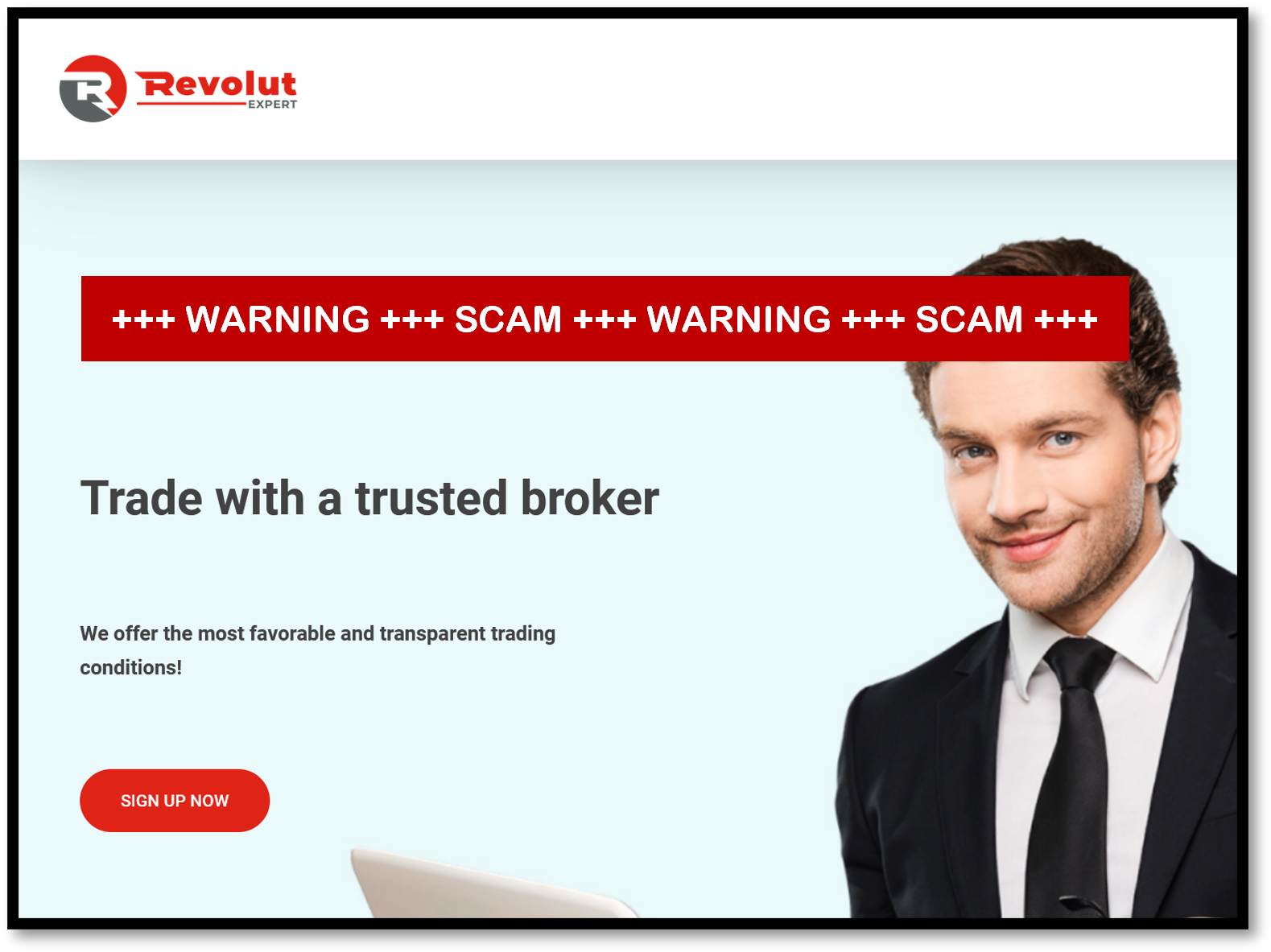
We find that our final model, CheXbert, is able to outperform the previous best rules-based labeler with statistical significance, setting a new SOTA for report labeling on one of the largest datasets of chest x-rays. We demonstrate superior performance of a biomedically pretrained BERT model first trained on annotations of a rulebased labeler and then finetuned on a small set of expert annotations augmented with automated backtranslation. In this work, we introduce a BERT-based approach to medical image report labeling that exploits both the scale of available rule-based systems and the quality of expert annotations.
#JINGPAI LABEL EXPERT MANUAL#
Existing approaches to report labeling typically rely either on sophisticated feature engineering based on medical domain knowledge or manual annotations by experts. The extraction of labels from radiology text reports enables large-scale training of medical imaging models.
#JINGPAI LABEL EXPERT LICENSE#
License from us (For Commercial Purposes): Abstract CheXbert: Combining Automatic Labelers and Expert Annotations for Accurate Radiology Report Labeling Using BERTĬheXbert is an accurate, automated deep-learning based chest radiology report labeler that can label for the following 14 medical observations: Fracture, Consolidation, Enlarged Cardiomediastinum, No Finding, Pleural Other, Cardiomegaly, Pneumothorax, Atelectasis, Support Devices, Edema, Pleural Effusion, Lung Lesion, Lung Opacity
